AI vs ML vs Analytics
Artificial Intelligence (AI), Machine Learning (ML), and Analytics are three distinct yet interconnected concepts that have become increasingly popular in the world of technology. While they share some similarities, it is important to understand their differences and how they can be applied in various industries. This article aims to shed light on AI, ML, and Analytics, highlighting their key features and applications.
Key Takeaways:
- AI, ML, and Analytics are different but related concepts in the world of technology.
- AI focuses on creating intelligent systems that can mimic human decision-making processes.
- ML is a subset of AI that enables systems to learn and improve from experience without being explicitly programmed.
- Analytics involves analyzing data to gain insights and make data-driven decisions.
The Difference Between AI, ML, and Analytics
Artificial Intelligence (AI) is the broadest concept of the three, encompassing the development of intelligent systems that can perform tasks typically requiring human intelligence. AI systems are designed to simulate human decision-making processes and often incorporate elements of ML and Analytics to achieve their goals.
Machine Learning (ML) is a subset of AI that focuses on algorithms and statistical models that enable systems to learn and improve from experience without explicit programming. ML algorithms can identify patterns in data and make predictions or decisions based on those patterns.
Analytics, on the other hand, is the practice of analyzing data to gain insights and make data-driven decisions. It involves the use of various techniques, such as statistical analysis and data mining, to extract meaningful information from data. Analytics can be applied in diverse fields, including marketing, finance, healthcare, and more.
Applications of AI, ML, and Analytics
The applications of AI, ML, and Analytics are vast and continue to expand across numerous industries. From personal digital assistants to self-driving cars, these technologies are transforming various aspects of our lives.
For instance, in the healthcare industry, AI can be utilized to accurately diagnose medical conditions and suggest appropriate treatment plans, potentially revolutionizing patient care.
ML plays a crucial role in recommendation systems, where it analyzes user behavior and past interactions to suggest personalized recommendations for products, movies, or songs. These algorithms power platforms like Netflix and Spotify, enhancing user experience and engagement.
AI, ML, and Analytics in Business
In the business world, AI, ML, and Analytics are becoming key drivers of growth and innovation. Organizations are leveraging these technologies to uncover hidden patterns in data, automate processes, and gain a competitive edge.
Companies are increasingly using Analytics to gain insights into customer behavior, market trends, and operational efficiency. By analyzing large volumes of data, businesses can make informed decisions, optimize processes, and improve overall profitability.
Moreover, AI and ML are being integrated into customer service operations, allowing companies to provide personalized experiences and faster response times for their customers.
Comparing AI, ML, and Analytics
Artificial Intelligence (AI) | Machine Learning (ML) | Analytics | |
---|---|---|---|
Definition | Building intelligent systems that mimic human decision-making processes. | Enabling systems to learn and improve from experience without explicit programming. | Analyzing data to gain insights and make data-driven decisions. |
Focus | Simulating human intelligence and decision-making. | Training models to make predictions or decisions based on patterns in data. | Extracting meaningful information from data for decision-making purposes. |
Applications | Self-driving cars, virtual assistants, healthcare diagnosis. | Recommendation systems, fraud detection, natural language processing. | Marketing analysis, risk assessment, demand forecasting. |
Conclusion
AI, ML, and Analytics are three interrelated concepts that have brought tremendous advancements to various industries. While AI focuses on simulating human intelligence, ML enables systems to learn and improve from experience, and Analytics involves analyzing data to make informed decisions. Together, these technologies have the potential to revolutionize the way we live, work, and interact with the world.
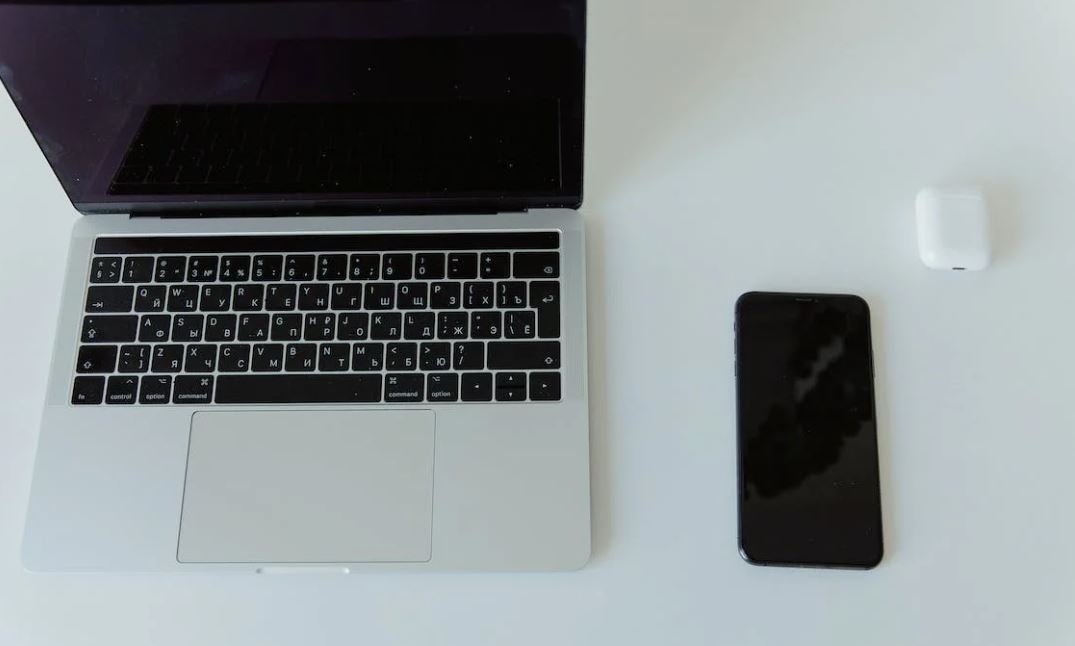
Common Misconceptions
AI
One common misconception people have around AI is that it is essentially the same as machine learning (ML) and analytics. While AI is a broader concept that encompasses ML and analytics, it is not the same as either of these two. AI refers to the development of computer systems that can perform tasks that would normally require human intelligence, such as learning, reasoning, and problem-solving.
- AI is not just about algorithms and data analysis.
- AI aims to replicate human cognitive abilities.
- AI includes subfields like natural language processing and computer vision.
ML
Another misconception is that ML and AI are interchangeable terms. While ML is a subset of AI, they are not synonymous. ML focuses on the development of algorithms and models that can enable computers to learn from data and make predictions or decisions. It is a method for achieving AI’s goal of reproducing human-like intelligence.
- ML involves training algorithms on data.
- ML algorithms can improve their performance over time.
- ML is used in various applications like image recognition and recommendation systems.
Analytics
Many people also mistakenly assume that analytics is equivalent to AI and ML. However, analytics is a separate field that involves the exploration, interpretation, and communication of data to uncover insights and support decision-making. Analytics often relies on statistical techniques and tools to extract meaning from data.
- Analytics involves data visualization and storytelling.
- Analytics helps businesses gain competitive advantages.
- Analytics encompasses descriptive, predictive, and prescriptive analytics.
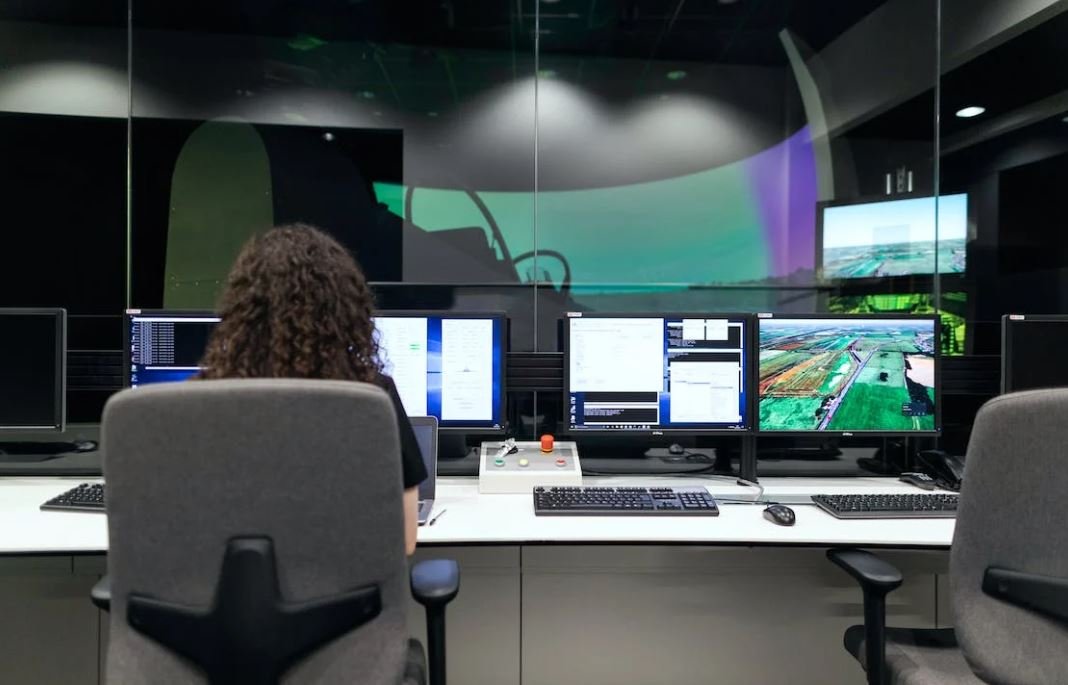
AI Applications in Everyday Life
Artificial Intelligence (AI) has become an integral part of our daily lives, impacting various industries and sectors. The following table highlights some incredible applications of AI that we often encounter:
Application | Description |
---|---|
Smart Assistants (e.g., Siri, Alexa) | AI-powered voice assistants that answer questions, perform tasks, and control smart devices. |
Self-Driving Cars | Vehicles equipped with AI technology to navigate, sense surroundings, and drive autonomously. |
Facial Recognition | AI algorithms identify and verify individuals based on their facial features. |
Virtual Personal Shoppers | AI-powered chatbots offer personalized product recommendations and assist customers in making purchase decisions. |
Medical Diagnosis Systems | AI-based software that aids doctors in diagnosing diseases by analyzing medical images, symptoms, and patient data. |
Distinguishing AI, ML, and Analytics
Although often used interchangeably, it is vital to understand the differences between Artificial Intelligence (AI), Machine Learning (ML), and Analytics. The following table depicts the unique characteristics and purposes of each:
Aspect | Artificial Intelligence (AI) | Machine Learning (ML) | Analytics |
---|---|---|---|
Definition | Simulates human intelligence and performs tasks that typically require human intelligence. | Utilizes algorithms and statistical models to enable computers to learn and improve from experience. | Examines data to uncover insights and trends that aid in decision-making. |
Focus | Mimick human intelligence and perform tasks autonomously. | Train algorithms to learn patterns from data and make accurate predictions or decisions. | Analyze data to gain insights and provide information for improved decision-making. |
Examples | Siri, self-driving cars, facial recognition systems. | Recommendation systems, fraud detection algorithms, speech recognition. | Business intelligence, market research, predictive modeling. |
Goal | To create systems that can think, learn, and self-improve. | To design computer programs that can learn from data and improve performance. | To utilize data analysis to optimize decision-making and improve processes. |
Impact of AI on Job Market
Artificial Intelligence (AI) is revolutionizing the job market, introducing new possibilities and transforming employment landscapes. The table below showcases the predicted impact of AI on various industries:
Industry | Impact of AI |
---|---|
Transportation | Self-driving vehicles are expected to replace long-haul truck drivers. |
Retail | Automated checkout systems and AI-powered inventory optimization will streamline operations and reduce the need for cashiers. |
Healthcare | AI-driven diagnosis systems will enhance accuracy and efficiency, impacting the roles of radiologists and pathologists. |
Manufacturing | Robotic automation will lead to a reduction in manual labor and the need for assembly line workers. |
Education | AI tutors and personalized learning platforms may change the role of teachers, offering tailored education to students. |
Machine Learning Algorithms in Action
Machine Learning (ML) algorithms are incredibly versatile, with various types designed to handle specific tasks. Here are some ML algorithms and their applications:
Algorithm | Application |
---|---|
Neural Networks | Recognizing patterns in images, speech, and text. |
Random Forest | Classifying spam emails, predicting customer churn. |
Support Vector Machines | Image recognition, sentiment analysis. |
K-means Clustering | Market segmentation, anomaly detection. |
Reinforcement Learning | Training autonomous robots, game-playing AI. |
The Data Analytics Process
Data analytics involves a series of steps culminating in valuable insights. The following table outlines the key stages of the data analytics process:
Stage | Description |
---|---|
Data Collection | Gathering relevant and reliable data from various sources. |
Data Cleaning | Preprocessing and removing errors or inconsistencies in the data. |
Data Exploration | Examining and visualizing data to discover patterns and correlations. |
Data Analysis | Applying statistical methods and algorithms to gain insights. |
Data Visualization | Presenting data visually through graphs, charts, and dashboards. |
Uses of Predictive Analytics
Predictive analytics utilizes historical data and statistical algorithms to make predictions about future events. The table below highlights some common uses of predictive analytics:
Use Case | Application |
---|---|
Customer Churn Prediction | Identifying customers who are likely to leave a service or product. |
Financial Forecasting | Predicting stock market trends or estimating future sales. |
Risk Assessment | Evaluating the likelihood of specific events occurring or potential risks in different scenarios. |
Weather Forecasting | Anticipating weather patterns and extreme events. |
Recommendation Systems | Providing personalized product or content recommendations based on user preferences. |
Machine Learning in Healthcare
Machine Learning (ML) is transforming the healthcare industry, revolutionizing diagnosis, treatment, and patient care. The following table illustrates the impact of ML in healthcare:
Application | Description |
---|---|
Medical Image Analysis | Using ML algorithms to detect anomalies in X-rays, MRI scans, and histopathology images. |
Predictive Medicine | Utilizing ML models to forecast disease progression, treatment outcomes, and potential complications. |
Drug Discovery | Accelerating the exploration of potential drugs through virtual screening and predictive modeling. |
Personalized Healthcare | Designing tailored treatment plans based on patient-specific data and characteristics. |
Healthcare Chatbots | Providing virtual assistance, triaging symptoms, and offering medical advice. |
Challenges in AI Adoption
While the potential of Artificial Intelligence (AI) is vast, its adoption faces various challenges. The table below outlines some hurdles in implementing AI:
Challenge | Description |
---|---|
Data Privacy | Ensuring the protection of personal data and maintaining privacy standards. |
Ethical Considerations | Addressing concerns regarding AI decision-making, bias, and potential unintended consequences. |
Workforce Adaptation | Preparing the workforce for AI integration and potential job displacement. |
Algorithmic Transparency | Understanding and explaining the decision-making process of complex AI algorithms. |
Technological Limitations | Overcoming constraints such as computational power, data availability, and algorithmic accuracy. |
Conclusion
Artificial Intelligence (AI), Machine Learning (ML), and Analytics are revolutionizing our world, enhancing everyday life and transforming industries. AI applications, ranging from smart assistants to self-driving cars, have become ubiquitous. Distinct from each other, AI simulates human intelligence, ML focuses on training algorithms, while analytics leverages data for decision-making. As AI advances, it significantly impacts the job market and poses challenges relating to privacy, ethics, adaptation, transparency, and technology. With the continuous development and integration of these technologies, the future holds immense opportunities for innovation and growth.
AI vs ML vs Analytics
Frequently Asked Questions
What is the difference between AI, ML, and Analytics?
Artificial Intelligence (AI) is a broad concept that encompasses a range of technologies that enable machines to simulate human intelligence. Machine Learning (ML) is a subset of AI that focuses on the ability of machines to learn and improve from experience. Analytics, on the other hand, refers to the process of extracting insights and making informed decisions based on data analysis.
How does AI work?
AI systems work by analyzing large amounts of data, recognizing patterns, and making predictions or decisions based on that analysis. This is done using techniques such as machine learning, natural language processing, and computer vision.
What is Machine Learning?
Machine Learning is a subset of AI that focuses on the development of algorithms and statistical models that enable machines to learn and make predictions or decisions without being explicitly programmed. ML models are trained on large amounts of data to identify patterns and make accurate predictions or decisions.
How does Machine Learning differ from traditional programming?
In traditional programming, explicit rules and instructions are provided for the machine to follow. In contrast, in Machine Learning, algorithms are trained on data to learn patterns and make decisions. ML models are capable of improving their performance over time as they gather more data and learn from it.
What is the role of Analytics in AI and ML?
Analytics plays a crucial role in AI and ML by providing insights and actionable information based on data analysis. Analytics helps in understanding patterns, identifying trends, making predictions, and optimizing algorithms in AI and ML systems.
What are the applications of AI?
AI has a wide range of applications across various industries. Some common applications include natural language processing, computer vision, recommendation systems, autonomous vehicles, fraud detection, and healthcare diagnostics.
Is AI just about robots?
No, AI is not just about robots. While robots are one application of AI, there are many other applications that do not involve physical robots. AI technologies can be used in software applications, data analysis, predictive modeling, and more.
How can AI and ML benefit businesses?
AI and ML can benefit businesses in various ways. They can automate repetitive tasks, improve operational efficiency, enhance customer experience, enable predictive maintenance, optimize pricing strategies, and provide valuable insights for decision-making.
What skills are required for working with AI and ML?
Working with AI and ML requires a combination of technical and analytical skills. These may include programming skills (Python, R, etc.), data analysis, statistics, machine learning algorithms, and problem-solving skills. A strong understanding of mathematics and computer science fundamentals is also beneficial.
What is the future of AI, ML, and Analytics?
The future of AI, ML, and Analytics is promising. These fields are evolving rapidly, and advancements in technology are driving new opportunities and applications. AI and ML are expected to continue transforming industries and enhancing various aspects of our lives, while analytics will continue to play a critical role in extracting valuable insights from data.