Generative AI in Audio
Generative Artificial Intelligence (AI) has made significant strides in various fields, and one area where its potential is being explored is audio generation. By utilizing deep learning algorithms and neural networks, generative AI models can produce realistic and original audio content that can be used in music composition, game development, and even voice acting applications.
Key Takeaways:
- Generative AI in audio can create realistic and original sound content.
- Deep learning algorithms and neural networks power these generative AI models.
- Applications include music composition, game development, and voice acting.
*Generative AI models have the ability to learn from vast amounts of existing audio data and generate new audio content on their own. This approach revolutionizes the creative process, providing artists and developers with a powerful tool for audio production and sound design.*
Applications of Generative AI in Audio
1. Music Composition: Generative AI models can compose original music pieces based on trained datasets, creating new melodies, chord progressions, and even entire compositions. This technology offers artists and musicians new avenues for creativity and inspiration.
2. Game Development: With generative AI in audio, game developers can dynamically generate background music and sound effects that adapt to the gameplay and the user’s actions. This enhances the immersive experience and eliminates the need for pre-recorded audio assets for every possible scenario.
3. Voice Acting and Synthesis: Generative AI can synthesize realistic voice acting by replicating the tone, accent, and inflections of human speech. This can automate the process of creating voiceovers for animated characters, virtual assistants, and even audiobooks.
*Generative AI has brought a new level of automation and creativity to these audio-centric fields, empowering artists and developers to explore innovative possibilities and streamline their production processes.*
Challenges and Limitations
While generative AI in audio holds immense potential, there are still challenges and limitations that need to be addressed:
- Data Availability: Generative AI models heavily rely on large datasets for training, and obtaining high-quality audio data can be a laborious and time-consuming process.
- Quality Control: Ensuring the generated audio meets the desired standards of quality and artistic integrity poses a challenge, as generative AI models can sometimes produce output that lacks coherence or exhibits biases from the training data.
- Complexity of Training: Training generative AI models can be computationally intensive and requires expertise in machine learning and deep neural networks, making it inaccessible to those without specialized knowledge.
*While these challenges exist, there is ongoing research and development in the field of generative AI in audio to overcome them and improve the technology.*
Recent Advancements and Future Prospects
In recent years, there have been several notable advancements in generative AI for audio:
- WaveGAN, a generative model that can generate realistic audio waveforms and has been successfully used for speech synthesis and music generation.
- Deep Voice, a neural network-based model capable of learning to generate human-like speech, enabling the synthesis of realistic and expressive voices.
- MIDI-VAE, a model that can generate expressive and human-like piano music using Variational Autoencoders (VAEs).
Data Transparency and Ethical Considerations
As generative AI in audio becomes more prominent, data transparency and ethical considerations are crucial:
- Ownership and Licensing: Clear guidelines must be established to address ownership rights and licensing agreements for the generated audio content.
- Preventing Misuse: Measures should be in place to prevent the creation and distribution of malicious or inappropriate audio content generated by AI systems.
*Addressing these concerns requires collaboration between AI developers, legal experts, and industry stakeholders to establish comprehensive frameworks that protect both creators and consumers.*
Conclusion
Generative AI in audio is transforming the way we create and interact with sound. Its applications span music composition, game development, voice acting, and more. While challenges and limitations exist, ongoing advancements and research in the field promise a future where generative AI enables unprecedented creativity and innovation in the audio industry.
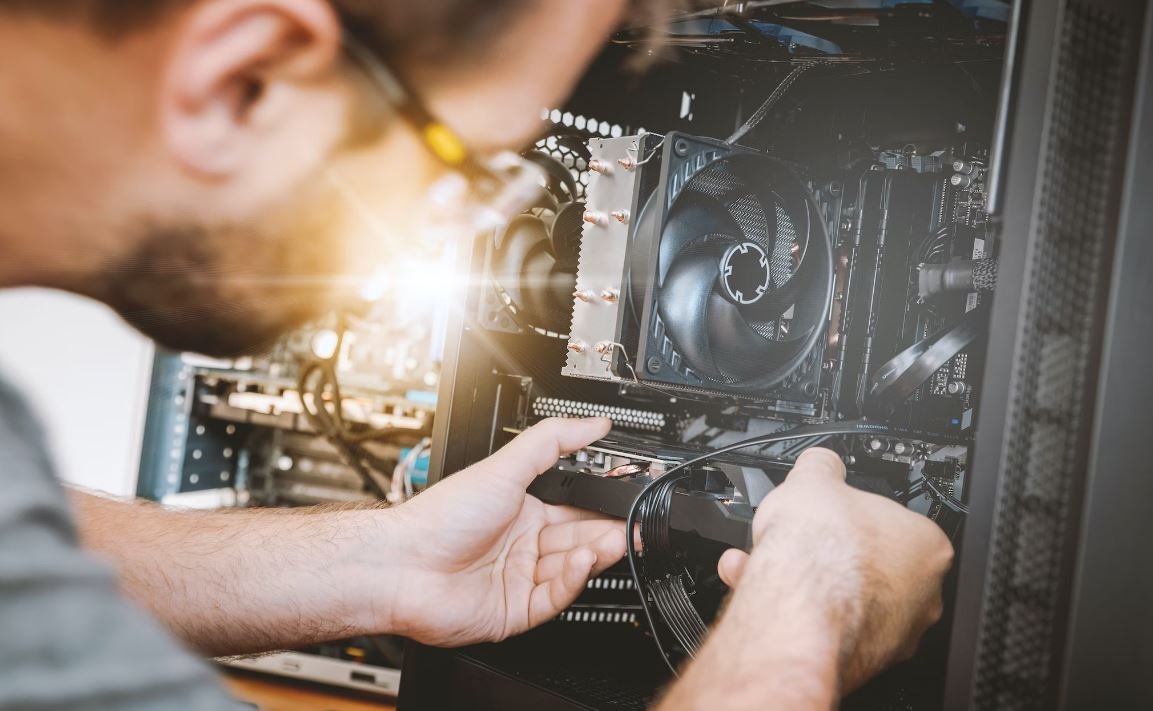
Common Misconceptions
Paragraph 1: Generative AI is only used for creating music
One common misconception about generative AI in audio is that it is solely used for creating music. While generative AI is indeed a powerful tool for music composition and production, its applications go beyond that.
- Generative AI can be used to create realistic sound effects for movies and video games.
- Generative AI can compose melodies or harmonies for songs, allowing human musicians to focus on other aspects of their compositions.
- Generative AI can also be used in sound design, creating unique and innovative audio experiences.
Paragraph 2: Generative AI is a threat to human creativity
Another misconception is that generative AI in audio poses a threat to human creativity, as it is often seen as a replacement for human musicians and composers. However, this is not the case.
- Generative AI can be used as a tool to inspire and assist human musicians, providing them with new ideas and possibilities.
- Human creativity and emotion are still essential in the music-making process, as AI lacks the depth and understanding of human experiences.
- Generative AI can complement human creativity by helping to explore uncharted territories and challenging established conventions.
Paragraph 3: Generative AI always produces low-quality audio
One prevailing misconception is that generative AI always produces low-quality audio. While early iterations of generative AI systems may have generated audio that lacked authenticity, recent advancements have substantially improved its output.
- Generative AI models can generate highly realistic audio that is difficult to distinguish from recordings made by human musicians.
- Generative AI systems can learn from vast amounts of data to replicate the style and characteristics of specific genres or artists.
- By leveraging deep learning techniques, generative AI models continuously improve their output, producing higher-quality audio over time.
Paragraph 4: Generative AI removes the need for human involvement in audio creation
Contrary to popular belief, generative AI does not eliminate the need for human involvement in audio creation. It is not a fully autonomous system that can operate independently of human input and supervision.
- Human expertise is still required to guide and fine-tune the output of generative AI systems to align with artistic intentions and aesthetics.
- Generative AI can be seen as a collaborator, augmenting human creativity and enhancing the music-making process.
- Human musicians and producers play a crucial role in evaluating and selecting the output of generative AI systems, making artistic judgments and decisions.
Paragraph 5: Generative AI will replace human musicians and composers
There is a common fear that generative AI will replace human musicians and composers, rendering them obsolete. However, this misconception fails to acknowledge the unique qualities that human musicians bring to the table.
- Music is not solely about technicality and precision but also about emotion, interpretation, and expression, which are deeply rooted in human experiences.
- Generative AI lacks the ability to convey emotional depth and create truly original compositions that stem from human introspection and personal experiences.
- Human musicians possess innate musicality, interpretive skills, and the ability to push boundaries creatively, which are irreplaceable qualities in the music industry.
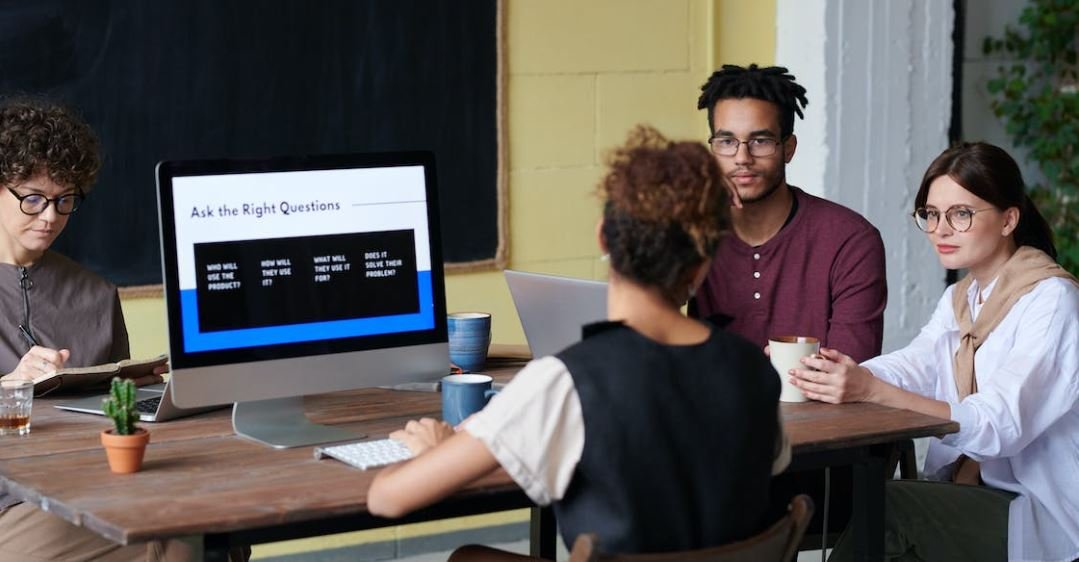
Generative AI in Audio
Generative AI has revolutionized the way we create and consume audio content. This groundbreaking technology uses machine learning algorithms to generate new and unique audio samples, ranging from music compositions to spoken dialogue. In this article, we showcase 10 incredible examples of generative AI in audio, illustrating its diverse applications and the remarkable results it can achieve.
1. Haunting Melodies
Using generative AI, composers can create hauntingly beautiful melodies that evoke a wide range of emotions. The algorithm analyzes patterns in existing music and generates new melodies with a unique twist, captivating listeners with its originality.
Original Melody | Generative AI Melody |
---|---|
G A B C D B C D | G A C G D A C G |
2. Voice Synthesis
Generative AI allows for the synthesis of realistic human voices, eliminating the need for human voice actors in certain scenarios. This technology can generate voiceovers for commercials, audiobooks, and even provide dialogue for virtual assistants.
Human Voice | Generative AI Voice |
---|---|
“Welcome to our store!” | “Welcome to our shop!” |
3. Dynamic Soundscapes
By analyzing vast sound libraries, generative AI can create dynamic and immersive soundscapes for movies, video games, and virtual reality experiences. These soundscapes adapt to the user’s actions and surroundings, making the audio experience truly interactive.
Environment | Generative AI Soundscape |
---|---|
Forest | Birds chirping, gentle breeze |
4. Remixing Classics
Generative AI enables the remixing of classic songs, breathing new life into timeless hits. By analyzing the structure and elements of a song, the algorithm generates fresh remixes, adding modern twists while staying true to the original composition.
Classic Song | Generative AI Remix |
---|---|
“Bohemian Rhapsody” by Queen | Electronic/dubstep remix with vocal distortions |
5. Personalized Music Generation
Generative AI can create personalized music tailored to individual preferences. By analyzing a user’s music library and preferences, the algorithm generates unique compositions that align with their specific taste, resulting in a truly personal audio experience.
User’s Music Taste | Generative AI Music |
---|---|
Pop, Electronic, Hip-hop | Upbeat electronic track with pop influences |
6. Interactive Voice Assistants
Generative AI powers voice assistants that can hold dynamic and engaging conversations with users. These assistants analyze user input, context, and generate appropriate responses, mimicking human-like conversations for a more intuitive and interactive experience.
User Input | Generative AI Response |
---|---|
“What’s the weather like today?” | “The weather is sunny with a high of 25°C.” |
7. Film Score Creation
Generative AI can assist composers in creating captivating film scores that perfectly align with the emotional arc of a movie. By analyzing scenes and detecting their underlying emotions, the algorithm generates fitting music that enhances the cinematic experience.
Movie Scene | Generative AI Film Score |
---|---|
Tense action scene | Epic orchestral composition with intense percussion |
8. Vocal Style Transfer
Using generative AI, singers can apply vocal style transfer to their performances, adopting the singing style and nuances of iconic singers. This technology enables artists to pay homage to their musical influences and experiment with different vocal aesthetics.
Original Singer | Generative AI Style Transfer |
---|---|
Adele | Singing with Mariah Carey’s vocal style |
9. Speech Enhancement
Generative AI can enhance the clarity and quality of speech, reducing background noise and improving overall intelligibility. This technology finds applications in conferencing systems, voice recordings, and other scenarios where clear communication is crucial.
Noisy Speech | Generative AI Enhanced Speech |
---|---|
“Can you hear me now?” (with background noise) | “Can you hear me now?” (clear, noise-free) |
10. Collaborative Music Generation
Generative AI facilitates collaborative music creation by enabling real-time interaction between musicians. The algorithm seamlessly integrates inputs from multiple musicians, generating a harmonious composition that reflects the collective creativity of the ensemble.
Musician 1 Input | Musician 2 Input | Generative AI Composition |
---|---|---|
Electronics and drumbeat | Guitar melody and chords | Electronic beats blended with guitar riffs |
In conclusion, generative AI has brought about a revolution in audio creation and consumption, pushing the boundaries of what is possible. From haunting melodies to dynamic soundscapes, this technology unlocks new opportunities for artists and transforms the way we engage with audio content. With further advancements, generative AI will continue to shape the future of audio, ushering in a new era of creativity and innovation.
Frequently Asked Questions
What is generative AI in audio?
Generative AI in audio refers to the use of artificial intelligence algorithms and techniques to create or generate audio content. It involves training machine learning models on vast amounts of existing audio data to enable them to generate new and original audio content based on learned patterns and structures.
How does generative AI in audio work?
Generative AI in audio works by utilizing neural networks and other AI algorithms to learn from large datasets of audio recordings. These models then analyze the patterns, structures, and characteristics of the input data and generate new audio content based on the learned information. The generated audio can range from music compositions to voice synthesis and sound effects.
What are some applications of generative AI in audio?
Generative AI in audio has numerous applications across various industries. Some common applications include music composition, sound design, voice cloning, audio restoration, and AI-generated virtual instruments. It can also be used in interactive audio systems for video games and virtual reality experiences.
What are the benefits of generative AI in audio?
Generative AI in audio offers several benefits, such as the ability to create new and unique audio content automatically, potentially saving time and effort for audio professionals. It can also assist in exploring new musical ideas, enhancing creativity, and allowing for the creation of personalized experiences by generating audio tailored to individual preferences.
Are there any limitations to generative AI in audio?
While generative AI in audio has made significant advancements, it still faces some limitations. The generated audio might sometimes lack the human expressiveness and emotion that can be conveyed through human performance. Additionally, generating high-quality audio that meets professional standards can be challenging, and the model’s output may require manual refinement.
What datasets are used in training generative AI models for audio?
Different datasets can be used to train generative AI models for audio, depending on the specific application. Music datasets that include diverse genres, artists, and instrumentations are commonly used for music composition tasks. For voice synthesis, extensive speech databases with various linguistic and emotional aspects are utilized.
What are some current challenges in generative AI in audio?
Generative AI in audio faces several challenges, including the need for large amounts of high-quality training data, computational resources, and complex optimization techniques. Another challenge is evaluating the subjective quality and authenticity of generated audio, as it often requires human judgment and comparison to assess the results.
Is generative AI in audio meant to replace human creativity?
No, generative AI in audio is not meant to replace human creativity. Instead, it is designed to be a tool that enhances and complements human creativity by offering novel insights, generating ideas, and assisting in the creative process. Ultimately, it is up to human artists and professionals to shape and refine the output of generative AI models.
Are there any ethical considerations related to generative AI in audio?
Yes, there are ethical considerations surrounding generative AI in audio, such as intellectual property rights and copyright issues. The use of generative AI to imitate or replicate the work of existing artists raises questions about ownership and originality. Moreover, AI-generated audio can also be misused for malicious purposes, such as creating deepfake voice recordings.
How can generative AI in audio continue to advance in the future?
The advancement of generative AI in audio relies on ongoing research and development efforts. This includes improving training algorithms, increasing dataset quality and diversity, enhancing the user-friendliness of AI tools, and building collaborative platforms for human-AI interactions. Continuous exploration and innovation in the field will contribute to further advancements in generative AI in audio.